Who Is Fact-Checking the “Fact-Checkers”? Oh Wait, That’s My Job
Crusing social media can be like being a storm chasing racing down the road after a tornado. You never know when you’re going hit some bull.
I’m tired of these self-anointed “Fact-Checkers” who believe they, among all of humanity, hold the key to special knowledge. I read their so-called “Fact Check” articles, and it’s really too easy to blow holes in their reasoning.
So I grabbed one, at random, from this guy, and found that I could debunk his debunking of people posting that Wolff had found an increase in coronavirus infection following the flu shot. So I gave his article a rating:
“UNSUPPORTED”.
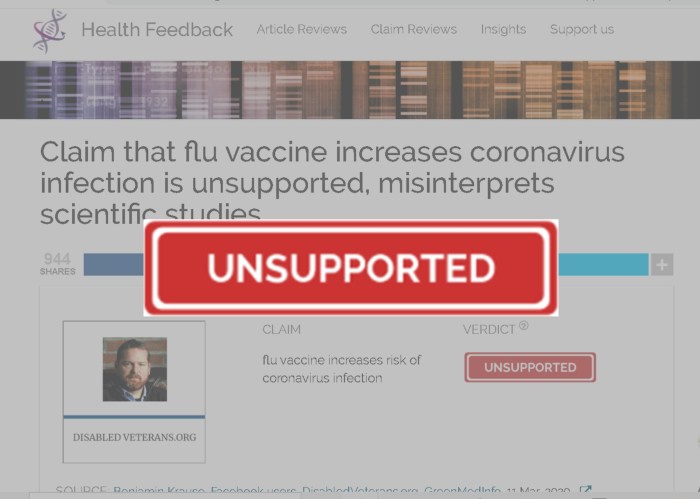
It took me about 30 seconds to find the flaws in his reasoning. I’ll share one: you cannot “Fact Check” based on opinion, so “Misinterprets scientific studies” only means “varies from my opinion”. That’s a shallow slice, but I’m just warming up…
This so-called “Fact-Checked” cites an article that analyzes the same data. The study he cites is Sworonski et al. (2020). The first flaw in their study was to consider people who had been vaccinated <2 weeks before symptoms as “unvaccinated”. This is capricious and arbitrary. Why? Because they are vaccinated. Their second flaw was obtuse inclusion criteria; they arbitrarily report that “Fever was not required for adults aged 65 years and older after 2010–2011.” Why? What about before then? This leads to a heterogenous population, adding variation unaccounted for in the sample groups. If I had reviewed the study I would have insisted on at least an internally consistent set of inclusion criteria!
So why did this so-called “Fact Checker” not criticize the study that he cited for these obvious flaws?
Oh wait, I’m not done.
There is a further flaw, this one fatal, and very well known, in the Sworonski et al., study: Healthy User Bias. According to HUB, people who are not vaccinating may be less healthy than those who do not vaccinate because they cannot tolerate vaccines as well… so they may also be more prone to more showing symptoms leading to a respiratory viral infection diagnosis, utterly confusing causality.
But wait, there’s more…
The largest the problem with observational studies? Most of the time, boils down to how one chooses to design the study and analyze the data.
Skowronski et al. has the gall to criticize Wolff for including test-positive patients in the control group.
“Wolff retained influenza test-positive specimens in NIRV test-negative control groups, thereby violating the core prerequisite for valid TND analysis.”
Here’s their reasoning:
“In assessing Wolff’s paper we identified a major methodological problem to account for his unexpected findings… In combined NIRV analysis, relative to pan-negative controls, Wolff adjusted for age and excluded specimens that tested influenza positive. In that analysis, shown in his Table 3, the OR approached unity, indicating no vaccine effect as expected. Conversely, in univariate (unadjusted) analysis of individual NIRV outcomes (eg, coronaviruses), Wolff retained influenza test-positive specimens in NIRV test-negative control groups, thereby violating the core prerequisite for valid TND analysis. In the context of effective influenza vaccine, influenza cases would have a lower likelihood of vaccination; as such, their inclusion would systematically reduce the proportion vaccinated in the control group and thereby inflate ORs comparing vaccine exposure between NIRV cases and controls. (emphasis mine). We illustrate the impact of this bias in Supplementary Material 3, where we have reanalyzed Wolff’s data as well as our own, comparing influenza vaccine effect against NIRV when influenza test-positive specimens are properly excluded (as per TND prerequisite) or improperly included (as per Wolff [4]) within the control group. In both datasets and for all NIRVs, ORs for influenza vaccination are biased higher when influenza cases are erroneously included in the control group.“
Wait. Just. A. Freaking. Minute.
Test-negative design of the efficacy of influenza includes the knowledge of the whether a person is infected with influenza, or not, so as to get at an idealized estimate of the efficacy of the influenza vaccine, NOT to drive other analyses such as whether a person who (unknowingly, asymptomatically) has the flu virus, gets vaccinated anyway, and then as consequence a weakened or confused immune system, gets another respiratory viral infection. That’s safety. Oops.
Sowolski et al. are therefore 100% wrong to criticize an re-interpret Wolff.
I want to say I’m done here, and mic drop, but not… just… yet.
Test-Negative Study Designs Are Irrelevant for Clinical Reality
In fact, the vaccinologists have really made a seriously bad decision to use Test-Negative study design to estimate flu vaccine efficacy althogther. Why?
#TranslationalFailure
Translational failure is when a study is conducted that is supposed to inform on a given clinical question for a specific clinical population, but via its study design, is render irrelevant due to the problem of non-representativeness of the sample groups for the clinical populations of interest.
The only population for which a test-negative study design, or any clinical study design is relevant is the study design that matches the actual clinical practice.
Thus, when we find a population where people are tested for the flu virus first, and are THEN vaccinated (or not), the test-negative study designs of flu vaccine efficacy MIGHT then be more relevant to reality.
Test negative study designs are uniquely irrelevant to reality regarding flu vaccine efficacy because they may enrich the study for those people who are likely to have a positive response to the flu vaccine, or for those people who are less likely to experience viral interference due to being vaccinated while infected with a dissimilar influenza type. TNDs have nothing to do with real estimates of influenza vaccine efficacy in the real world.
So, next time Facebook or other social media outlets cite an alleged “Fact-Checker” as showing that this study or the other does not show what the authors of the study themselves concluded (as in the case of Wolff), you just run right over that bull, take it as a badge of honor (if it’s your post) and share this blog article in the comment of your post. You can find it at #FactCheckingGetsReal anytime you need it.
Maybe if we always share this back, Facebook censors will learn a thing or two about clinical trial study design before they accept the baloney being served by the self-annointed keepers of the #Truth.
Time to being tagged by a Fact Checker in 10, 9, 8, 7…
James Lyons-Weiler
Allison Park, PA
8.27.2020
This article first appeared on jameslyonsweiler.com.